Tue 24 September 2013
This article introduces a python module for summarizing geospatial raster datasets based on vector geometries (i.e. zonal statistics).
A common task in many of my data workflows involves "zonal statistics"; summarizing raster data based on vector geometries. Despite many
alternatives (starspan, the QGIS Zonal Statistics plugin, ArcPy and R) there
were none that were
- open source
- fast enough
- flexible enough
- worked with python data structures
We'd written a wrapper around starspan for madrona (see madrona.raster_stats ) but
relying on shell calls and an aging, unmaintained C++ code base was not cutting
it.
So I set out to create a solution using numpy, GDAL and python. The
rasterstats
package was born.
`python-raster-stats` on github
Example
Let's jump into an example. I've got a polygon shapefile of continental US
state boundaries and a raster dataset of annual precipitation from the
North American Environmental
Atlas.
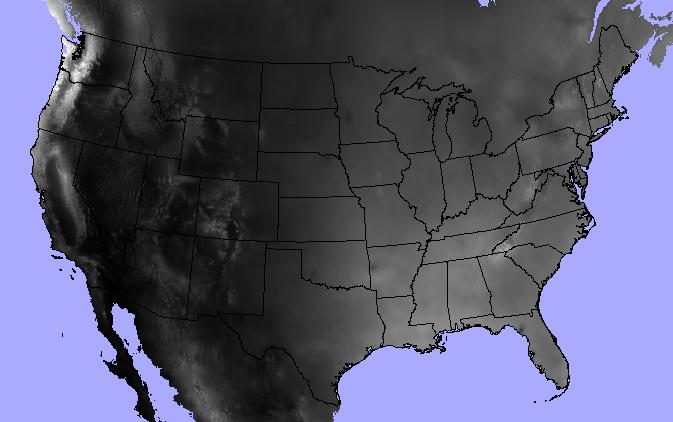
states = "data/boundaries_contus.shp"
precip = "data/precipitation.tif"
The raster_stats
function is the main entry point. Provide a vector and a
raster as input and expect a list of dicts, one for each input feature.
from rasterstats import raster_stats
rain_stats = raster_stats(states, precip, stats="*", copy_properties=True)
len(rain_stats) # continental US; 48 states plus District of Columbia
49
Print out the stats for a given state:
[x for x in rain_stats if x['NAME'] == "Oregon"][0]
{'COUNTRY': 'USA',
'EDIT': 'NEW',
'EDIT_DATE': '20060803',
'NAME': 'Oregon',
'STATEABB': 'US-OR',
'Shape_Area': 250563567264.0,
'Shape_Leng': 2366783.00361,
'UIDENT': 124704,
'__fid__': 35,
'count': 250510,
'majority': 263,
'max': 3193.0,
'mean': 779.2223903237395,
'median': 461.0,
'min': 205.0,
'minority': 3193,
'range': 2988.0,
'std': 631.539502512283,
'sum': 195203001.0,
'unique': 2865}
Find the three driest states:
[(x['NAME'], x['mean']) for x in
sorted(rain_stats, key=lambda k: k['mean'])[:3]]
[('Nevada', 248.23814034118908),
('Utah', 317.668743027571),
('Arizona', 320.6157232064074)]
And write the data out to a csv.
from rasterstats import stats_to_csv
with open('out.csv', 'w') as fh:
fh.write(stats_to_csv(rain_stats))
Geo interface
The basic usage above shows the path of an entire OGR vector layer as the first argument. But raster-stats
also supports other vector features/geometries.
- Well-Known Text/Binary
- GeoJSON string and mappings
- Any python object that supports the geo_interface
- Single objects or iterables
In this example, I use a geojson-like python mapping to specify a single geometry
geom = {'coordinates': [[
[-594335.108537269, -570957.932799394],
[-422374.54395311, -593387.5716581973],
[-444804.1828119133, -765348.1362423564],
[-631717.839968608, -735441.9510972851],
[-594335.108537269, -570957.932799394]]],
'type': 'Polygon'}
raster_stats(geom, precip, stats="min median max")
[{'__fid__': 0, 'max': 1011.0, 'median': 451.0, 'min': 229.0}]
Categorical
We're not limited to descriptive statistics for continuous rasters either; we
can get unique pixel counts for categorical rasters as well. In this example,
we've got a raster of 2005 land cover (i.e. general vegetation type).
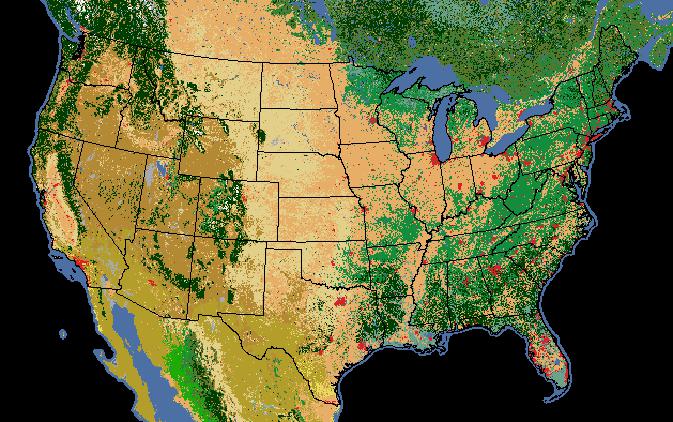
Note that
we can specify only the stats that make sense and the categorical=True
provides a count of each pixel value.
>>> landcover = "/data/workspace/rasterstats_blog/NA_LandCover_2005.img"
>>> veg_stats = raster_stats(states, landcover,
stats="count majority minority unique",
copy_properties=True,
nodata_value=0,
categorical=True)
>>> [x for x in veg_stats if x['NAME'] == "Oregon"][0]
{1: 999956,
3: 6,
5: 3005,
6: 198535,
8: 2270805,
10: 126199,
14: 20883,
15: 301884,
16: 17452,
17: 39246,
18: 28872,
19: 2174,
'COUNTRY': 'USA',
'EDIT': 'NEW',
'EDIT_DATE': '20060803',
'NAME': 'Oregon',
'STATEABB': 'US-OR',
'Shape_Area': 250563567264.0,
'Shape_Leng': 2366783.00361,
'UIDENT': 124704,
'__fid__': 35,
'count': 4009017,
'majority': 8,
'minority': 3,
'unique': 12}
Of course the pixel values alone don't make much sense. We need to interpret the
pixel values as land cover classes:
Value, Class_name
1 Temperate or sub-polar needleleaf forest
2 Sub-polar taiga needleleaf forest
3 Tropical or sub-tropical broadleaf evergreen
4 Tropical or sub-tropical broadleaf deciduous
5 Temperate or sub-polar broadleaf deciduous
6 Mixed Forest
7 Tropical or sub-tropical shrubland
8 Temperate or sub-polar shrubland
9 Tropical or sub-tropical grassland
10 Temperate or sub-polar grassland
11 Sub-polar or polar shrubland-lichen-moss
12 Sub-polar or polar grassland-lichen-moss
13 Sub-polar or polar barren-lichen-moss
14 Wetland
15 Cropland
16 Barren Lands
17 Urban and Built-up
18 Water
19 Snow and Ice
So, for our Oregon example above we can see that, despite Oregon's reputation as
a lush green landscape, the majority land cover class (#8) is "Temperate or sub-
polar shrubland" at 2.27m pixels out of 4 millions total.
There's a lot more functionality that isn't covered in this post but you get the
picture... please check it out and let me know what you think.